SEO Header
As insurers navigate a rapidly evolving market, machine learning redefines everything from underwriting to claims processing to customer service. This groundbreaking technology enables insurers to predict risks with unparalleled precision, streamline operations, and deliver personalized customer experiences.
Let’s look at the applications, benefits, and challenges of machine learning in insurance.
What is Machine Learning for Insurance?
Machine learning, a subset of artificial intelligence (AI), allows systems to learn from data and improve performance without explicit programming. In insurance, machine learning automates the analysis of vast datasets to identify patterns, predict risks, and enable data-driven decision-making. Learning from historical and real-time data, machine learning algorithms empower insurers to anticipate trends, accurately price policies, and improve operational efficiency.
One way to think of it is like this: in the past, underwriters relied on manual methods to evaluate risks — spreadsheets, rules of thumb, and years of experience. Today, machine learning takes those traditional approaches to the next level, using advanced algorithms to assess risks faster and more precisely.
Data from claims histories, environmental factors, economic trends, and even IoT devices all feed into ML models, enabling insurers to make faster and smarter decisions.
Applications and Benefits of Machine Learning in the Insurance Industry
The applications of machine learning in insurance are vast and impactful. ML touches nearly every aspect of the insurance process, from underwriting to fraud detection. Let’s dig into some of the most transformative insurance machine learning use cases, each contributing to improved risk management and customer satisfaction.
Underwriting and Risk Assessment
Machine learning enhances underwriting and risk management by automating data analysis and generating nuanced policyholder insights. Traditional underwriting relied on historical averages and basic demographic data. Machine learning, by contrast, analyzes millions of data points — including real-time data from connected devices — to produce precise risk profiles and policy prices.
For example, an insurer specializing in commercial property coverage can use ML to analyze historical claims data, weather patterns, and property maintenance records. The result? More tailored pricing and faster policy issuance. Not only does this save time, but it also ensures better relationships with policyholders by providing a level of customization they’ve come to expect.
Claims Processing and Fraud Detection
Few areas benefit from machine learning like claims processing and fraud prevention. ML streamlines claims workflows by automating routine tasks and reducing human errors in processes like document verification and claims triaging. Insurers can use predictive analytics to identify potential delays and optimize claims resolutions.
Detection of fraud cases, a perennial challenge for insurers, has also been transformed by machine learning. Anomaly detection algorithms can use claims data to flag unusual patterns or behaviors. For instance, if an auto repair shop files identical invoices for multiple claims, an ML model can raise a red flag for further investigation of just that particular case.
Customer Service and Personalization
Modern customers expect insurers to meet their needs quickly and precisely. Machine learning tools such as chatbots, virtual assistants, and recommendation engines are helping insurers meet this challenge.
- Chatbots and virtual assistants handle routine inquiries, allowing human agents to focus on complex cases.
- Recommendation engines suggest policies, answer common questions, and guide users through policy options while tailoring them to individual preferences and circumstances.
- Sentiment analysis gauges customer satisfaction in real-time, enabling insurers to address issues proactively.
Consider a business owner visiting an insurer’s website to explore coverage options. An ML-powered chatbot could analyze their browsing behavior, predict their needs, and then recommend the most relevant policies, significantly improving the user experience.
Predictive Analytics and Risk Forecasting
Cutting across most of the other benefits, predictive analytics is one of the most powerful applications of machine learning in the insurance industry. Whether it's assessing the likelihood of a natural disaster, predicting a customer’s claim frequency, or anticipating market shifts, predictive models provide actionable insights to mitigate risks before they arise.
By analyzing historical claims data, environmental factors, and even social trends, ML models help insurers anticipate future risks. For instance, an insurer specializing in natural disaster coverage can use machine learning to predict the likelihood of hurricanes or floods in specific regions. This allows them to adjust pricing, recommend preventive measures to policyholders, and minimize losses.
Challenges of Implementing Machine Learning and Insurance Processes
Despite the numerous benefits of machine learning, insurance companies face a number of challenges in implementing this technology. Here, we outline some main obstacles insurers face when adopting ML solutions.
Data Quality and Privacy Concerns
Machine learning models are only as good as the data they’re trained on. However, insurers often struggle with incomplete, inaccurate, or biased data, which can affect the performance of ML models.
Additionally, privacy regulations like GDPR and CCPA have made it more challenging for insurers to manage customer data. They must ensure that they are collecting relevant data, safeguarding it, and complying with privacy laws. Balancing the need for data with privacy concerns is an ongoing challenge for insurers adopting ML.
Integration with Legacy Systems
Some insurers still operate on legacy systems, which can complicate integrating modern ML solutions. Legacy systems may not support the data processing requirements of data required for ML models, and making the transition to newer systems can be costly and time-consuming.
To address these challenges, insurers can adopt a phased approach to integration. They gradually migrate to more advanced systems while still maintaining their current infrastructure, allowing them to leverage the benefits of ML without disrupting their ongoing operations.
Regulatory and Ethical Concerns
Insurance is undoubtedly among the most highly regulated industries. Integrating machine learning into insurance processes requires careful consideration of regulations like CCPA, as we’ve seen. Insurers must also ensure their ML models are transparent, fair, and comply with industry standards. Ethical concerns, such as the risk of bias in algorithms, need to be addressed.
Insurers must adopt transparent processes that explain their models' algorithmic decision-making processes to ensure fairness and that customers understand how their data is used.
Innovations and Advanced Techniques in Insurance Machine Learning
The rapid evolution of machine learning is transforming the insurance industry. It’s not just reshaping traditional insurance practices — it’s pushing the boundaries of what’s possible in this data-driven era. As machine learning continues to evolve, insurers leverage advanced techniques to push those boundaries further.
Natural Language Processing (NLP)
Natural language processing (NLP) allows insurers to analyze unstructured data, such as customer emails, claims forms, and social media interactions. By processing this data, ML algorithms can extract valuable insights that improve customer service and claims processing.
For instance, NLP tools can automatically categorize claims and determine their urgency based on the language used in the reports. This capability helps streamline the claims process and improve the efficiency of customer interactions.
Deep Learning and Neural Networks
Deep learning, a subset of machine learning, represents the cutting edge of ML. It involves neural networks that can analyze complex data with multiple processing layers. Deep learning in insurance can more accurately assess complex risks, detect anomalies in claims data, or even analyze images for damage assessments.
Real-Time Data Processing and IoT Integration
Integrating the Internet of Things (IoT) with machine learning enables insurers to access real-time data from devices like smart home sensors and wearable technology. This data helps insurers offer personalized policies, such as usage-based insurance, where premiums are adjusted based on real-time insights. Smart devices could, for example, detect potential water leaks or fire hazards, allowing insurers to alert policyholders to mitigate risks before they escalate.
The Future of Machine Learning in Insurance
As machine learning matures, its impact on the insurance industry will only grow, resulting in more sophisticated, data-driven, and automated processes. Below are some key trends shaping the future ML and AI use cases in insurance.
Increased Adoption and Investment
The future of machine learning in insurance promises even greater innovation and efficiency. According to the KPMG Global Tech Report 2023, 52% of companies identify AI, including machine learning, as the top technology for achieving their goals in the next three years. Moreover, 59% of companies across various industries exploring or deploying AI have accelerated their rollout investment in this technology.
Enhanced Underwriting and Risk Assessment
Next-generation AI and machine learning algorithms will continue revolutionizing underwriting by enabling insurers to analyze more complex datasets. AI-driven underwriting will improve the accuracy of risk assessments, allowing insurers to develop more detailed customer profiles and refine their pricing strategies. Transforming traditional underwriting from a largely manual process will result in a dynamic, data-driven process that adapts in real-time to emerging risks and market conditions.
Improved Operational Efficiency
AI technologies could generate up to $1.1 trillion in annual value for the global insurance industry, according to McKinsey. This remarkable figure includes approximately $400 billion from enhanced pricing, underwriting, and promotion technologies, plus an additional $300 billion through AI-powered customer service and personalized offerings.
These gains stem from streamlined workflows, automated routine tasks, and more accurate decision-making processes that reduce operational costs while improving service quality.
Advanced Fraud Detection
The future of fraud detection in insurance lies in sophisticated anomaly detection systems that operate with increasing autonomy and precision. These systems will analyze patterns across vast networks of claims data, identifying potential fraud in real-time before losses occur.
Combining traditional fraud indicators with advanced behavioral analytics will help insurers spot sophisticated fraud schemes that might otherwise go undetected, leading to substantial cost savings and increased trust.
Regulatory and Ethical Considerations
Building on the regulatory and ethical challenges we discussed earlier, the future landscape will likely see the emergence of specialized frameworks specifically designed for AI and ML governance in insurance. Insurers must prepare for increased scrutiny of their AI-driven decisions, particularly in underwriting and claims processes.
Regulatory requirements focused on clearly documenting machine learning models' decision-making processes and regular audits to prevent algorithmic bias can be expected. This evolution will push insurers to develop more transparent and fair systems that comply with legal requirements and regulations and proactively address the ethical concerns surrounding AI and ML. Doing so is likely to build long-term trust with both customers and regulators.
Stay Up to Date on Machine Learning’s Impact on the Insurance Industry
Machine learning in insurance is no longer a futuristic concept — it’s here and transforming how insurers operate. A critical tool for innovation, ML enables smarter risk assessment, enhances customer interactions, and improves overall operational efficiency. As the technology continues to improve, the benefits ML insights offer will only expand, offering insurers a competitive edge in a rapidly evolving market.
Staying informed about the technological developments in machine learning is crucial for insurance professionals. Keep ahead of the curve and subscribe to Insurance Insider US for comprehensive insights and expert analysis on the latest trends shaping the P&C insurance market.
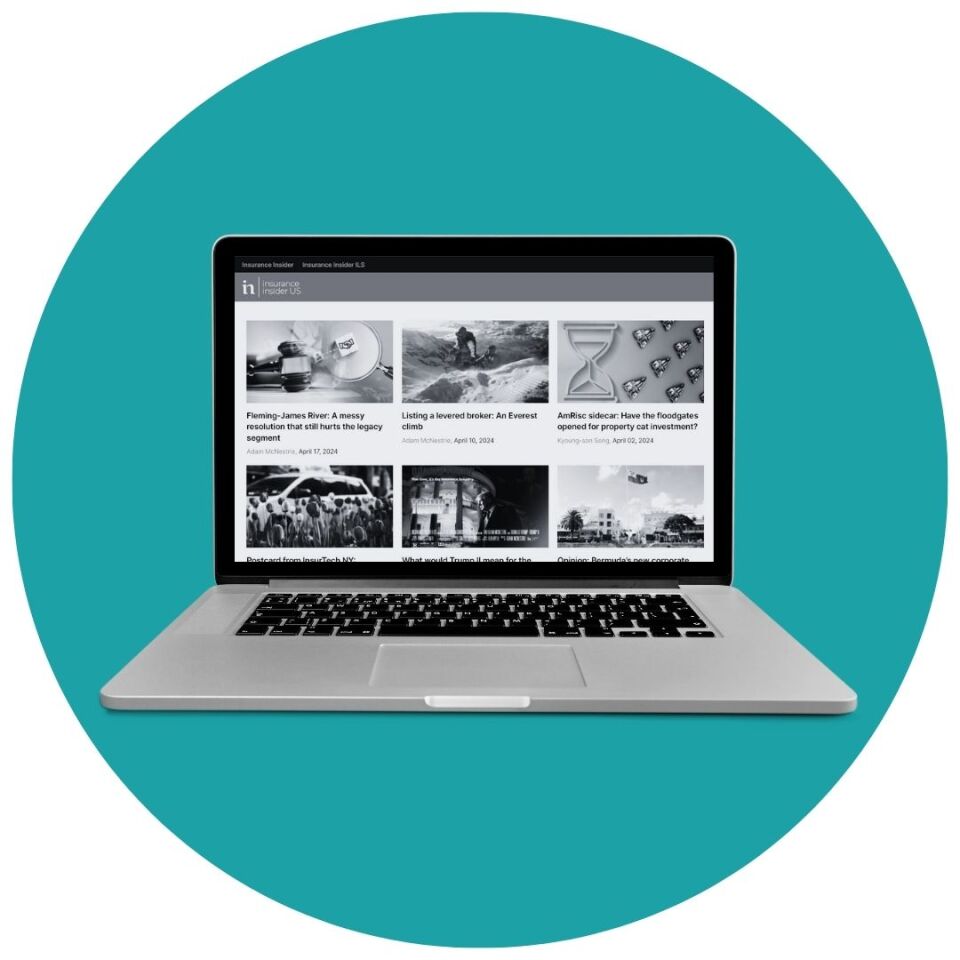
Subscribe to Insurance Insider US today and harness the insights needed to drive your business forward.
You'll get:
- Breaking news alerts and digests
- In-depth analysis and opinion
- Research you won't find anywhere else
- Tools mapping M&A and talent data
- Subscriber discounts to events
- Access to an esteemed reporting team