Header
The insurance industry has always been about managing risks, forecasting outcomes, and preparing for the unknown. Historically, insurers relied on experience, intuition, and limited data to inform these decisions. But times have changed. Today, predictive analytics is stepping in as the driving force behind smarter, faster, and more efficient decision-making.
From underwriting to fraud detection, the intersection of predictive analytics and insurance enables property and casualty (P&C) insurers to gain a competitive edge in the market. A 2023 Forbes study found that predictive analytics improved operational efficiency by a staggering 60%, while customer experience improved by 95%.
In this feature, we’re digging into what predictive analytics means for insurers, how it’s impacting core functions, and why it’s a must-have tool in an industry driven by data and competition. We’ll also discuss challenges, future trends, and actionable steps to give you a headstart on your predictive analytics journey.
What is Predictive Analytics in Insurance?
Predictive analytics in insurance combines statistical techniques, machine learning models, and data analysis tools to anticipate future outcomes based on historical and real-time data. It forecasts potential risks, claims, customer behaviors, and market trends.
Predictive analytics is a game-changer in the P&C insurance sector because it moves insurers from reactive decision-making to proactive planning. Instead of waiting for risks or losses to unfold, insurers anticipate them and take preemptive action.
For example, predictive analytics allows insurers to assess risk more accurately during underwriting, optimize premium pricing based on customer behavior and market conditions, and detect and flag fraudulent claims. They can also identify high-risk areas for natural disasters or other catastrophic events and improve customer retention through better segmentation and personalization.
In short, predictive analytics takes the guesswork out of insurance and replaces it with proven techniques that yield actionable insights.
The Importance of Predictive Analytics for Insurers
Why is predictive analytics essential for insurers? The answer lies in its ability to address some of the industry’s biggest challenges while unlocking new opportunities.
- Tackling Growing Competition: The P&C insurance market is crowded, and insurers must stay ahead of competitors by delivering better products, faster service, and lower costs. Predictive analytics allows insurers to differentiate themselves by offering dynamic pricing, personalized products, and unparalleled customer experiences.
- Managing Increased Risks: From extreme weather events to political factors and cyber threats, insurers' risks are becoming more complex. Predictive models help insurers prepare for these uncertainties by analyzing vast amounts of data to identify patterns and forecast potential outcomes.
- Maximizing Profitability: Insurance profit margins are often razor-thin. Predictive analytics helps insurers protect their bottom line by improving underwriting accuracy, reducing fraud, and streamlining claims management.
Predictive analytics is critical for insurers in today’s hyper-competitive and data-driven market. Without this tool, you’ll lag behind the competition in your offerings and decision-making.
Key Predictive Analytics Techniques Used in Insurance
Insurance predictive analytics relies on a range of techniques to unlock the value hidden in data. Here are some of the most common methods used by insurance companies:
- Machine Learning (ML): Machine learning algorithms process massive datasets to uncover trends and relationships that traditional analysis might miss. These models continuously improve as they’re exposed to new data, making them invaluable for risk assessment and fraud detection.
- Regression Analysis: Regression analysis identifies the relationships between variables, such as the link between policyholder age and claims frequency. Insurers use this technique to forecast outcomes like loss probability or premium pricing.
- Decision Trees: Decision trees break down complex decisions into simple, logical steps. For instance, a decision tree might help an insurer determine whether a claim is legitimate or fraudulent based on specific triggers.
- Natural Language Processing (NLP): NLP tools analyze unstructured data, such as customer emails or claims descriptions, to identify patterns and insights. For example, NLP can flag inconsistencies in a claimant’s story that might indicate fraud.
Keep in mind that these techniques aren’t used in isolation. Instead, they’re combined to create robust predictive models that deliver actionable insights.
Core Applications of Predictive Analytics in the Insurance Industry
The insurance industry operates within a complex landscape, and P&C insurance providers, in particular, face the pressure of navigating competitive markets while managing unpredictable events and loss trends. Predictive analytics addresses these pain points by offering data-driven solutions with far-reaching applications across the insurance value chain.
Risk Assessment and Underwriting
Risk assessment lies at the heart of insurance operations. Predictive models analyze historical claims, demographic data, and environmental factors to assign risk scores accurately. This capability has elevated underwriting precision, allowing insurers to price policies competitively while avoiding overexposure to high-risk portfolios.
For example, insurers now use insurance predictive modeling to evaluate climate-related risks. By analyzing weather patterns and historical data, insurers can refine their underwriting models to better account for potential disasters.
Claims Management and Fraud Detection
Fraudulent claims cost insurers billions annually. Predictive analytics has proven invaluable in identifying suspicious patterns in claims data. For instance, machine learning algorithms can flag anomalies such as exaggerated losses or inconsistent policyholder histories, significantly reducing fraudulent payouts.
Moreover, claims processing times are reduced dramatically. By automating the analysis of claims data, predictive tools enable faster resolutions, enhancing customer satisfaction.
Customer Segmentation and Personalization
By leveraging predictive analytics, insurance companies get to understand their customers on a granular level. Insurers can deliver personalized products and proactive retention strategies by segmenting policyholders based on behaviors, risk profiles, and preferences.
An insurer might use predictive analytics to identify a segment of customers who are likely to lapse their policies. They could also create personalized offerings or reminders to improve retention rates, ultimately improving customer satisfaction and loyalty.
Pricing Optimization
Dynamic pricing, powered by predictive analytics, allows insurers to respond to real-time market trends and customer behaviors. By identifying patterns in purchasing behavior and risk appetite, insurers can price policies to balance competitiveness with profitability.
Disaster and Catastrophe Modeling
Natural disasters and catastrophic events pose significant risks to insurers. Predictive analytics aids in modeling these scenarios, helping insurers prepare for large-scale claims events. Advanced models use historical and environmental data to estimate potential losses and optimize coverage strategies.
Such models can show the potential impact of hurricanes, floods, or wildfires so that insurers can prepare financially for large-scale claims or develop risk mitigation strategies to protect policyholders.
Benefits of Predictive Analytics for Insurers
The strategic benefits of predictive analytics go well beyond operational improvements. Here’s why embracing this transformative technology is no longer optional but essential for sustained growth and resilience.
Improved Decision-Making and Risk Management
Predictive analytics empowers insurers to make data-driven decisions that minimize risks and optimize operations. Insurers get a clearer picture of potential risks, enabling them to make informed decisions across all functions — from underwriting and claims management to customer retention and product development. Reduced uncertainty positions insurers to navigate complex market dynamics with more confidence.
Operational Efficiency and Cost Reduction
Automation powered by predictive tools eliminates inefficiencies in processes such as claims handling, underwriting, and customer service. Streamlining these operations helps companies reduce costs without compromising service quality.
Enhanced Customer Experience
Today’s customers expect fast and personalized service — predictive analytics helps insurers deliver just that. By anticipating customer needs and offering tailored solutions, insurers can build stronger relationships and foster loyalty. For example, predictive models can identify when customers risk canceling their policy and trigger a retention campaign to address their concerns.
Challenges in Implementing Predictive Analytics in Insurance
While the benefits of predictive analytics are clear, implementing this technology isn’t without its challenges. Insurers must address several barriers to unlock its full potential.
Data Quality and Integration Issues
Data quality and integration are among the most critical challenges in predictive analytics. Insurers often manage data from diverse sources, including policyholder records, claims histories, and third-party providers. This data is frequently fragmented, outdated, or inconsistent, making it difficult to support accurate predictive modeling.
To overcome this challenge, insurers must prioritize data cleaning, validation, and integration efforts. Leveraging advanced data management platforms and building pipelines that unify disparate datasets can help establish a reliable foundation. For example, insurers could implement real-time data validation processes or adopt ETL (Extract, Transform, Load) tools to streamline data flow.
Regulatory and Compliance Constraints
The insurance industry operates within a complex regulatory environment. Insurance regulatory compliance laws, such as GDPR and CCPA, impose strict guidelines on collecting, storing, and analyzing customer data. Insurers must balance the power of predictive analytics with the ethical and legal responsibilities of data usage.
Non-compliance with these regulations can result in significant financial penalties and damage to brand reputation. To mitigate this risk, insurers should integrate compliance frameworks into their predictive analytics strategies. Training teams on privacy laws, employing data anonymization techniques, and collaborating with legal advisors during model development could help overcome this challenge.
Skill Gaps and Organizational Buy-In
Implementing predictive analytics requires specialized talent, including data scientists, machine learning engineers, and IT professionals. However, many companies face skill gaps in these areas, which can slow adoption and limit innovation. Beyond technical expertise, achieving organizational buy-in is equally critical. Resistance from leadership or operational teams can undermine efforts to integrate predictive tools effectively.
To bridge the skill gap, insurers should invest in talent development through training programs and partnerships with universities or analytics firms. Establishing a cross-functional team to oversee predictive initiatives ensures all stakeholders are aligned.
Future Trends in Predictive Analytics for Insurance
As insurers look to stay competitive, understanding emerging trends that will shape the future of predictive analytics in the insurance industry is crucial. So, what is soon to come for P&C carriers?
AI-Driven Predictive Analytics
Artificial intelligence is transforming predictive analytics by enhancing the accuracy and adaptability of models. Deep learning algorithms, for instance, can analyze vast datasets to uncover patterns that traditional methods might miss. Similarly, natural language processing (NLP) enables insurers to extract insights from unstructured data sources such as customer reviews, social media posts, and claims narratives.
Real-Time Data and IoT
The proliferation of IoT devices offers insurers unprecedented access to real-time data that can transform predictive analytics. Devices such as industrial sensors, connected building systems, and supply chain trackers generate continuous data streams invaluable for proactive risk management. Insurers can use this information to predict equipment failures, mitigate workplace hazards, or optimize logistics operations.
Connected sensors in commercial properties could, for instance, monitor environmental conditions, detecting potential issues like overheating machinery or water damage risks. By acting on these insights, insurers minimize claims and help clients reduce operational risks.
Generative AI in Underwriting and Claims
Generative AI, a subset of artificial intelligence, is beginning to make waves in insurance just like it is in other industries. Unlike traditional predictive models, which analyze historical data, generative AI can simulate potential scenarios, enabling insurers to evaluate risks and opportunities more comprehensively.
Decision-making can be enhanced by generative AI models, which analyze historical claims data to foresee the likelihood and impact of future catastrophic events. In underwriting, through gen AI predictive modeling, insurance providers can create hypothetical risk profiles based on different combinations of variables, helping insurers test the impact of new policies or changes in coverage.
In claims management, it can simulate potential claim outcomes, allowing adjusters to prioritize cases and refine their decision-making overall.
Getting Started with Predictive Analytics in Insurance
Implementing predictive analytics in insurance requires careful planning and strategic investment. Many insurers succeed by launching focused pilot projects in specific departments, such as claims or underwriting, before expanding across their organization. Equally important is assembling a skilled team capable of harnessing these tools. It may involve upskilling current staff or partnering with analytics vendors and seeking consultancy services to develop and integrate predictive solutions effectively.
From improved risk assessment and fraud detection to personalized customer experiences and optimized pricing, the applications of predictive modeling in insurance are far-reaching. By embracing this transformative technology, insurers can make data-driven decisions, improve their operational efficiency, and ultimately, solidify their position as market leaders.
For more comprehensive insights into how predictive analytics transforms our industry, subscribe to Insurance Insider US and stay ahead of the curve.
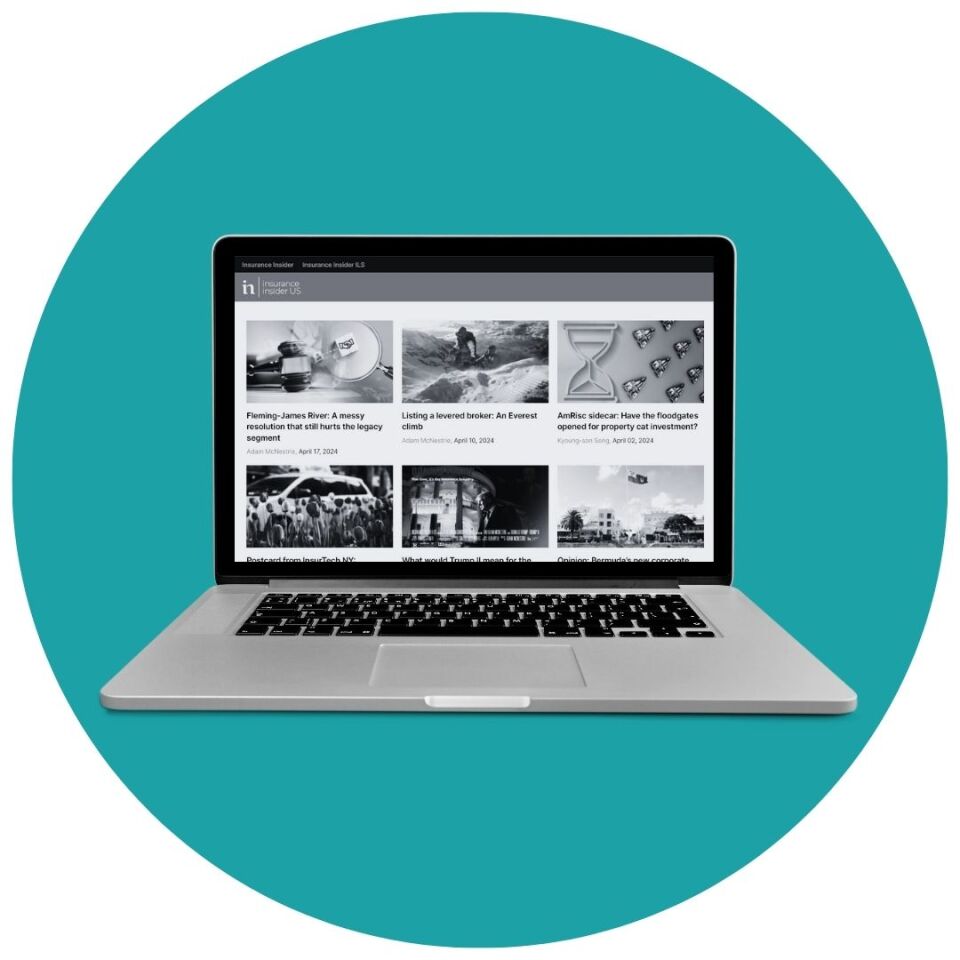
Subscribe to Insurance Insider US today and harness the insights needed to drive your business forward.
You'll get:
- Breaking news alerts and digests
- In-depth analysis and opinion
- Research you won't find anywhere else
- Tools mapping M&A and talent data
- Subscriber discounts to events
- Access to an esteemed reporting team